Two superconducting qubits acting as giant artificial atoms. These “atoms” are protected from decoherence yet still interact with each other through the waveguide.
Courtesy of the researchers
Michaela Jarvis | MIT News correspondent
MIT researchers have introduced a quantum computing architecture thatcan perform low-error quantum computations while also rapidly sharing quantum information between processors. The work represents a key advance toward a complete quantum computing platform.
Previous to this discovery, small-scale quantum processors have successfully performed tasks at a rate exponentially faster than that of classical computers. However, it has been difficult to controllably communicate quantum information between distant parts of a processor. In classical computers, wired interconnects are used to route information back and forth throughout a processor during the course of a computation. In a quantum computer, however, the information itself is quantum mechanical and fragile, requiring fundamentally new strategies to simultaneously process and communicate quantum information on a chip.
“One of the main challenges in scaling quantum computers is to enable quantum bits to interact with each other when they are not co-located,” says William Oliver, an associate professor of electrical engineering and computer science, MIT Lincoln Laboratory fellow, and associate director of the Research Laboratory for Electronics. “For example, nearest-neighbor qubits can easily interact, but how do I make ‘quantum interconnects’ that connect qubits at distant locations?”
The answer lies in going beyond conventional light-matter interactions.
While natural atoms are small and point-like with respect to the wavelength of light they interact with, in a paper published today in the journal Nature, the researchers show that this need not be the case for superconducting “artificial atoms.” Instead, they have constructed “giant atoms” from superconducting quantum bits, or qubits, connected in a tunable configuration to a microwave transmission line, or waveguide.
This allows the researchers to adjust the strength of the qubit-waveguide interactions so the fragile qubits can be protected from decoherence, or a kind of natural decay that would otherwise be hastened by the waveguide, while they perform high-fidelity operations. Once those computations are carried out, the strength of the qubit-waveguide couplings is readjusted, and the qubits are able to release quantum data into the waveguide in the form of photons, or light particles.
“Coupling a qubit to a waveguide is usually quite bad for qubit operations, since doing so can significantly reduce the lifetime of the qubit,” says Bharath Kannan, MIT graduate fellow and first author of the paper. “However, the waveguide is necessary in order to release and route quantum information throughout the processor. Here, we’ve shown that it’s possible to preserve the coherence of the qubit even though it’s strongly coupled to a waveguide. We then have the ability to determine when we want to release the information stored in the qubit. We have shown how giant atoms can be used to turn the interaction with the waveguide on and off.”
An optical micrograph image of a chip with two superconducting qubits (yellow) acting as giant artificial atoms. Each giant atom connects to the waveguide (blue) at three distinct and well-separated locations.
Courtesy of the researchers
The system realized by the researchers represents a new regime of light-matter interactions, the researchers say. Unlike models that treat atoms as point-like objects smaller than the wavelength of the light they interact with, the superconducting qubits, or artificial atoms, are essentially large electrical circuits. When coupled with the waveguide, they create a structure as large as the wavelength of the microwave light with which they interact.
The giant atom emits its information as microwave photons at multiple locations along the waveguide, such that the photons interfere with each other. This process can be tuned to complete destructive interference, meaning the information in the qubit is protected. Furthermore, even when no photons are actually released from the giant atom, multiple qubits along the waveguide are still able to interact with each other to perform operations. Throughout, the qubits remain strongly coupled to the waveguide, but because of this type of quantum interference, they can remain unaffected by it and be protected from decoherence, while single- and two-qubit operations are performed with high fidelity.
“We use the quantum interference effects enabled by the giant atoms to prevent the qubits from emitting their quantum information to the waveguide until we need it.” says Oliver.
“This allows us to experimentally probe a novel regime of physics that is difficult to access with natural atoms,” says Kannan. “The effects of the giant atom are extremely clean and easy to observe and understand.”
The work appears to have much potential for further research, Kannan adds.
“I think one of the surprises is actually the relative ease by which superconducting qubits are able to enter this giant atom regime.” he says. “The tricks we employed are relatively simple and, as such, one can imagine using this for further applications without a great deal of additional overhead.”
Andreas Wallraff, professor of solid-state physics at ETH Zurich, says the research "investigates a piece of quantum physics that is hard or even impossible to fathom for microscopic objects such as electrons or atoms, but that can be studied with macroscopic engineered superconducting quantum circuits. With these circuits, using a clever trick, they are able both to protect their giant atom from decay and simultaneously to allow for coupling two of them coherently. This is very nice work exploring waveguide quantum electrodynamics."
The coherence time of the qubits incorporated into the giant atoms, meaning the time they remained in a quantum state, was approximately 30 microseconds, nearly the same for qubits not coupled to a waveguide, which have a range of between 10 and 100 microseconds, according to the researchers.
Additionally, the research demonstrates two-qubit entangling operations with 94 percent fidelity. This represents the first time researchers have quoted a two-qubit fidelity for qubits that were strongly coupled to a waveguide, because the fidelity of such operations using conventional small atoms is often low in such an architecture. With more calibration, operation tune-up procedures and optimized hardware design, Kannan says, the fidelity can be further improved.
Original article published on the MIT News website on July 29, 2020
News Image:
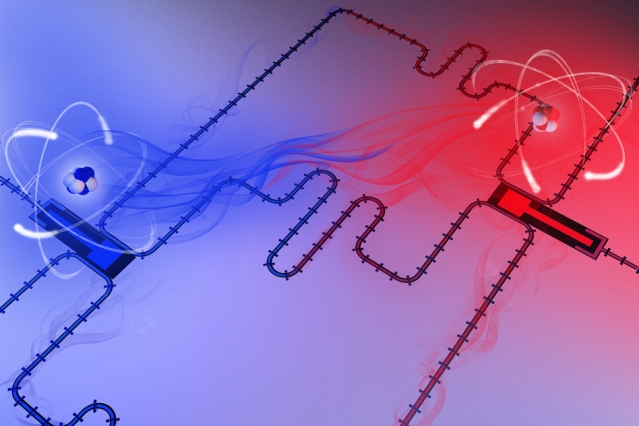