Faculty Positions at EECS
Two EECS students among those named Schwarzman Scholars
Kimberly Benard | Office of Distinguished Fellowships
Lisa Ho and Anita Liu will study for one year at Tsinghua University in China.
Left: Anita Liu ’17, Right: Lisa Ho ’17
Three undergraduate and one graduate student from MIT have been selected as Schwarzman Scholars. They are among the 129 members of the program’s class of 2018, who will pursue a year of study and leadership training at Tsinghua University in Beijing.
MIT’s four students, Alix de Monts ’14, Lisa Ho, Anita Liu, and Melody Liu, will study public policy, economics and business, and international studies, and spend a year immersed in an international community of thinkers, innovators, and senior leaders in business, politics, and society. Expenses for each scholar are fully funded by the program, which is housed at Tsinghua University.
Lisa Ho is a senior in computer science and mathematical economics. She plans to develop an international network of researchers dedicated to improving education, using economics and machine learning together to devise intelligent policy. She taught students in Oklahoma City and Boston, ran MIT’s Educational Studies Program (which annually teaches more than 3,000 students), developed engineering education software for Google, and examined principal management practices as a researcher for Harvard’s Department of Economics.
Anita Liu is majoring in computer science and engineering and in brain and cognitive sciences, with a minor in political science. She dreams of a world in which children are cared for and guided to achieve their full potential. She has participated in multiple policy internships on child care issues, and she is the executive director of a nonprofit dedicated to dual athletic-academic mentorship for underresourced Boston high school students. Through Schwarzman Scholars, she hopes to develop both leadership skills and a refined understanding of policy.
See the full list of recipients on MIT News.
News Image:
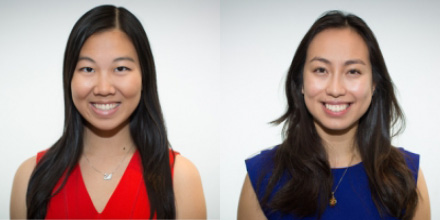
Learning words from pictures
Larry Hardesty | MIT News
System correlates recorded speech with images, could lead to fully automated speech recognition.
MIT researchers have developed a new approach to training speech-recognition systems that doesn’t depend on transcription. Instead, their system analyzes correspondences between images and spoken descriptions of those images, as captured in a large collection of audio recordings. Image: MIT News
Speech recognition systems, such as those that convert speech to text on cellphones, are generally the result of machine learning. A computer pores through thousands or even millions of audio files and their transcriptions, and learns which acoustic features correspond to which typed words.
But transcribing recordings is costly, time-consuming work, which has limited speech recognition to a small subset of languages spoken in wealthy nations.
At the Neural Information Processing Systems conference this week, researchers from MIT’s Computer Science and Artificial Intelligence Laboratory (CSAIL) are presenting a new approach to training speech-recognition systems that doesn’t depend on transcription. Instead, their system analyzes correspondences between images and spoken descriptions of those images, as captured in a large collection of audio recordings. The system then learns which acoustic features of the recordings correlate with which image characteristics.
“The goal of this work is to try to get the machine to learn language more like the way humans do,” says Jim Glass, a senior research scientist at CSAIL and a co-author on the paper describing the new system. “The current methods that people use to train up speech recognizers are very supervised. You get an utterance, and you’re told what’s said. And you do this for a large body of data.
“Big advances have been made — Siri, Google — but it’s expensive to get those annotations, and people have thus focused on, really, the major languages of the world. There are 7,000 languages, and I think less than 2 percent have ASR [automatic speech recognition] capability, and probably nothing is going to be done to address the others. So if you’re trying to think about how technology can be beneficial for society at large, it’s interesting to think about what we need to do to change the current situation. And the approach we’ve been taking through the years is looking at what we can learn with less supervision.”
Joining Glass on the paper are first author David Harwath, a graduate student in electrical engineering and computer science (EECS) at MIT; and Antonio Torralba, an EECS professor.
Visual semantics
The version of the system reported in the new paper doesn’t correlate recorded speech with written text; instead, it correlates speech with groups of thematically related images. But that correlation could serve as the basis for others.
If, for instance, an utterance is associated with a particular class of images, and the images have text terms associated with them, it should be possible to find a likely transcription of the utterance, all without human intervention. Similarly, a class of images with associated text terms in different languages could provide a way to do automatic translation.
Conversely, text terms associated with similar clusters of images, such as, say, “storm” and “clouds,” could be inferred to have related meanings. Because the system in some sense learns words’ meanings — the images associated with them — and not just their sounds, it has a wider range of potential applications than a standard speech recognition system.
To test their system, the researchers used a database of 1,000 images, each of which had a recording of a free-form verbal description associated with it. They would feed their system one of the recordings and ask it to retrieve the 10 images that best matched it. That set of 10 images would contain the correct one 31 percent of the time.
“I always emphasize that we're just taking baby steps here and have a long way to go,” Glass says. “But it’s an encouraging start.”
The researchers trained their system on images from a huge database built by Torralba; Aude Oliva, a principal research scientist at CSAIL; and their students. Through Amazon’s Mechanical Turk crowdsourcing site, they hired people to describe the images verbally, using whatever phrasing came to mind, for about 10 to 20 seconds.
For an initial demonstration of the researchers’ approach, that kind of tailored data was necessary to ensure good results. But the ultimate aim is to train the system using digital video, with minimal human involvement. “I think this will extrapolate naturally to video,” Glass says.
Merging modalities
To build their system, the researchers used neural networks, machine-learning systems that approximately mimic the structure of the brain. Neural networks are composed of processing nodes that, like individual neurons, are capable of only very simple computations but are connected to each other in dense networks. Data is fed to a network’s input nodes, which modify it and feed it to other nodes, which modify it and feed it to still other nodes, and so on. When a neural network is being trained, it constantly modifies the operations executed by its nodes in order to improve its performance on a specified task.
The researchers’ network is, in effect, two separate networks: one that takes images as input and one that takes spectrograms, which represent audio signals as changes of amplitude, over time, in their component frequencies. The output of the top layer of each network is a 1,024-dimensional vector — a sequence of 1,024 numbers.
The final node in the network takes the dot product of the two vectors. That is, it multiplies the corresponding terms in the vectors together and adds them all up to produce a single number. During training, the networks had to try to maximize the dot product when the audio signal corresponded to an image and minimize it when it didn’t.
For every spectrogram that the researchers’ system analyzes, it can identify the points at which the dot-product peaks. In experiments, those peaks reliably picked out words that provided accurate image labels — “baseball,” for instance, in a photo of a baseball pitcher in action, or “grassy” and “field” for an image of a grassy field.
In ongoing work, the researchers have refined the system so that it can pick out spectrograms of individual words and identify just those regions of an image that correspond to them.
“Possibly, a baby learns to speak from its perception of the environment, a large part of which may be visual,” says Lin-shan Lee, a professor of electrical engineering and computer science at National Taiwan University. “Today, machines have started to mimic such a learning process. This work is one of the earliest efforts in this direction, and I was really impressed when I first learned of it.”
“Perhaps even more exciting is just the question of how much we can learn with deep neural networks,” adds Karen Livescu, an assistant professor at the Toyota Technological Institute at the University of Chicago. “The more the research community does with them, the more we realize that they can learn a lot from big piles of data. But it is hard to label big piles of data, so it's really exciting that in this work, Harwath et al. are able to learn from unlabeled data. I am really curious to see how far they can take that.”
Read this article on MIT News.
Research Themes:
News Image:

Labs:
“Google Docs” for life sciences accelerates discovery
Rob Matheson | MIT News
Cloud-based platform assists in managing, sharing data facilitates research.
MIT spinout Benchling is bringing life science researchers a cloud-based platform that integrates different types of lab software into one place, with aims of making research and development easier, quicker, and more collaborative.
Numerous innovations have hit life science labs in the past decade, such as new techniques for designing DNA and editing genomes. Yet, researchers still rely on older tools — such as paper notebooks, Excel spreadsheets, and email — to manage data collected from those innovations. This means time is wasted organizing, finding, and duplicating information before even starting new experiments.
Now MIT spinout Benchling is bringing life science researchers a cloud-based platform that integrates different types of lab software into one place, with aims of making research and development easier, quicker, and more collaborative.
Using Benchling, researchers can design, record, analyze, share, and constantly update data in the cloud. Generated data can be shared with members of a team, and other scientists around the world. Benchling has, on average, increased scientist productivity by up 30 to 50 percent, automating organizational tasks and reducing experiment duplication, according to feedback from users, says MIT co-founder Saji Wickramasekara. “It really impacts the productivity and day-to-day life of researchers,” he says.
Wickramasekara and MIT alumnus Ashu Singhal ’11 invented the platform as a pet project to assist fellow researchers at the Institute. Now, Benchling — lauded by the media as the “Google Docs” for life sciences — is being used by more than 50,000 researchers at more than 1,000 research institutions worldwide, including by more than 600 students and professors at MIT.
“We built this from the ground up, combining the modules scientists use from day to day … into a simple, intelligent platform,” Wickramasekara says. “Ultimately, we want to empower scientists to further scale the pace of discovery.”
Making “living” data
Each year, pharmaceutical companies spend around $5 billion to bring one drug to market, and the industry spends roughly $150 billion on research and development through various programs. But a lot of gathered data must be manually updated and shared across various physical and digital resources, which wastes time and money.
Consider, for example, a biologist who wants to design and test new DNA pieces. Using traditional methods, the researcher plans the experiment on a paper notebook and an email calendar. Then, the researcher will use several different software packages to design DNA, analyze data, and document results.
None of those modules talk to each other; any changes made to data must be updated across all sources, “which sometimes doesn’t happen at all,” Wickramasekara says. Sometimes, in fact, valuable data goes missing or gets buried in spreadsheets or email attachments, he adds. And if scientists want to further collaborate on experiments, they generally exchange information via email, teleconferences, and meetings.
“Many scientists at times feel like glorified note-takers,” Wickramasekara says. “Due to the lack of integrated tool suites for life sciences researchers, [they] spend about 30 percent of their time flipping between different apps, figuring out how to share data that they’ve generated, and performing tasks that can be automated. That’s where Benchling steps in.”
Using Benchling, a biologist, for instance, will plan an experiment in a digital notebook, complete with integrated calendar entries and to-do lists. They then use an interface that combines 10 or more different molecular biology apps, such as a CRISPR guide design tool, plasmid visualizations, a sequence repository, and a genomic library to annotate DNA. After running tests in the lab, the biologist can document their work in a digital lab notebook, and everything syncs together. Scientists can later run detailed queries in a unique biological registration tool to, say, find samples below a specific pH level and their specific locations in the physical lab.
Increasingly, scientists are publishing academic papers with links to their data hosted on Benchling, Wickramasekara says. “Then they have this living document that, when corrections come in or they make adjustments, they add that new data and can keep one repository updated,” he says. “The data is not archived anymore — it’s living.”
Democratizing research
Today, Benchling users operate in the fields of synthetic biology, industrial biotechnology, genomics, gene therapy, antibody discovery, immuno-oncology, and others. They’re primarily from universities such as MIT, Stanford University, and Harvard University, as well as roughly 100 global biotech and pharmaceutical companies, such as Editas Medicine, Voyager Therapeutics, and Zymergen.
But Benchling aims, in the long term, to “democratize research,” Wickramasekara says. The plan is to add features that allow users to work, collaborate, and outsource experiments with contract research organizations, or CROs, which provide research services to the life-science labs, a common practice in the industry.
Scientists could, for instance, design an experiment in Benchling, outsource that experiment to CROs, and analyze and record the returned results in Benchling, all from their laptop. “That means research is no longer restricted to the wealthy and few — like just at MIT and Harvard, or the most well-resourced pharmaceutical companies,” Wickramasekara says. “Everyone [will have] access to the best possible labs in the world and can conduct experiments at the push of a button.”
Scratching the itch
Wickramasekara and Singhal initially came to MIT to study life sciences but soon turned to computer science and electrical engineering. In doing so, they observed the software industry had something the life sciences industry lacked: efficient online collaboration.
“In software, you can build a great product with a team of people from around the world. You have tools to collaborate and code effectively, and if some part of that process is suboptimal, an engineer somewhere will improve it, and the entire industry moves forward,” Wickramasekara says. “But the way that we work together on research [in life sciences] hasn’t really changed in the past decade.”
In 2012, they built a prototype for Benchling “to scratch our own itch” for making collaboration easier, Wickramasekara says. This first iteration, made strictly for DNA editing, was provided to a small number of researchers at MIT and Stanford. And it gained momentum: Graduate students saw it as a time-saver, while professors enjoyed collecting and archiving data, in one place, from a constant flow of students, Wickramasekara says. “We had a really passionate group of early adopters … and it grew from there,” he says.
In 2012, the startup was accepted into the YCombinator startup accelerator in Mountain View, California, and earned $900,000 in seed funding. The startup has raised a combined $13 million in funding since inception.
Over the years, Benchling has used the “number-one resource MIT has to offer, which is the people,” Wickramasekara says. Benchling’s first employees and investors, as well as many members of the current engineering team, hailed from MIT. “The company you build is as good as the team you recruit,” Wickramasekara says. “Being able to hit the ground running and hire so many super talented people from the MIT network was our key competitive advantage.”
Read this article on MIT News.
News Image:

Research Area:
Design your own custom drone
Adam Conner-Simons | MIT News
CSAIL system lets users design and fabricate drones with a wide range of shapes and structures.
A four-rotor "bunnycopter" developed at MIT's Computer Science and Artificial Intelligence Laboratory features propellers at different heights. Photo: Jason Dorfman/MIT CSAIL
This fall’s new Federal Aviation Administration regulations have made drone flight easier than ever for both companies and consumers. But what if the drones out on the market aren’t exactly what you want?
A new system from MIT’s Computer Science and Artificial Intelligence Laboratory (CSAIL) is the first to allow users to design, simulate, and build their own custom drone. Users can change the size, shape, and structure of their drone based on the specific needs they have for payload, cost, flight time, battery usage, and other factors.
To demonstrate, researchers created a range of unusual-looking drones, including a five-rotor “pentacopter” and a rabbit-shaped “bunnycopter” with propellers of different sizes and rotors of different heights.
“This system opens up new possibilities for how drones look and function,” says MIT Professor Wojciech Matusik, who oversaw the project in CSAIL’s Computational Fabrication Group. “It’s no longer a one-size-fits-all approach for people who want to make and use drones for particular purposes.”
The interface lets users design drones with different propellers, rotors, and rods. It also provides guarantees that the drones it fabricates can take off, hover and land — which is no simple task considering the intricate technical trade-offs associated with drone weight, shape, and control.
“For example, adding more rotors generally lets you carry more weight, but you also need to think about how to balance the drone to make sure it doesn’t tip,” says PhD student Tao Du, who was first author on a related paper about the system. “Irregularly-shaped drones are very difficult to stabilize, which means that they require establishing very complex control parameters.”
Du and Matusik co-authored a paper with PhD student Adriana Schulz, postdoc Bo Zhu, and Assistant Professor Bernd Bickel of IST Austria. It will be presented next week at the annual SIGGRAPH Asia conference in Macao, China.
Today’s commercial drones only come in a small range of options, typically with an even number of rotors and upward-facing propellers. But there are many emerging use cases for other kinds of drones. For example, having an odd number of rotors might create a clearer view for a drone’s camera, or allow the drone to carry objects with unusual shapes.
Designing these less conventional drones, however, often requires expertise in multiple disciplines, including control systems, fabrication, and electronics.
“Developing multicopters like these that are actually flyable involves a lot of trial-and-error, tweaking the balance between all the propellers and rotors,” says Du. “It would be more or less impossible for an amateur user, especially one without any computer-science background.”
But the CSAIL group’s new system makes the process much easier. Users design drones by choosing from a database of parts and specifying their needs for things like payload, cost, and battery usage. The system computes the sizes of design elements like rod lengths and motor angles, and looks at metrics such as torque and thrust to determine whether the design will actually work. It also uses an “LQR controller” that takes information about a drone’s characteristics and surroundings to optimize its flight plan.
One of the project’s core challenges stemmed from the fact that a drone’s shape and structure (its “geometry”) is usually strongly tied to how it has been programmed to move (its “control”). To overcome this, researchers used what’s called an “alternating direction method,” which means that they reduced the number of variables by fixing some of them and optimizing the rest. This allowed the team to decouple the variables of geometry and control in a way that optimizes the drone’s performance.
“Once you decouple these variables, you turn a very complicated optimization problem into two easy sub-problems that we already have techniques for solving,” says Du. He envisions future versions of the system that could proactively give design suggestions, like recommending where a rotor should go to accommodate a desired payload.
“This is the first system in which users can interactively design a drone that incorporates both geometry and control,” says Nobuyuki Umetani, a research scientist at Autodesk, Inc., who was not involved in the paper. “This is very exciting work that has the potential to change the way people design.”
The project was supported, in part, by the National Science Foundation, the Air Force Research Laboratory and the European Union’s Horizon 2020 research and innovation program.
Read this article on MIT News.
News Image:

Labs:
Four CSAIL researchers named ACM fellows
Rachel Gordon | MIT News
Association for Computer Machinery cites Erik Demaine, Fredo Durand, William Freeman, and Daniel Jackson for having "provided key knowledge" to computing.
Clockwise from top left: MIT professors Erik Demaine, Fredo Durand, Daniel Jackson, and William Freeman.
This week the Association for Computer Machinery (ACM) announced its 2016 fellows, which include four principal investigators from MIT’s Computer Science and Artificial Intelligence Laboratory (CSAIL): professors Erik Demaine, Fredo Durand, William Freeman, and Daniel Jackson. They were among the 1 percent of ACM members to receive the distinction.
“Erik, Fredo, Bill, and Daniel are wonderful colleagues and extraordinary computer scientists, and I am so happy to see their contributions recognized with the most prestigious member grade of the ACM,” says CSAIL Director Daniela Rus, who herself was named a fellow last year. “All of us at CSAIL are very proud of these researchers for receiving these esteemed honors.”
ACM’s 53 fellows for 2016 were named for their distinctive contributions spanning such computer science disciplines as computer vision, computer graphics, software design, machine learning, algorithms, and theoretical computer science.
“As nearly 100,000 computing professionals are members of our association, to be selected to join the top 1 percent is truly an honor,” says ACM President Vicki L. Hanson. “Fellows are chosen by their peers and hail from leading universities, corporations and research labs throughout the world. Their inspiration, insights and dedication bring immeasurable benefits that improve lives and help drive the global economy. ”
Demaine was selected for contributions to geometric computing, data structures, and graph algorithms. His research interests include the geometry of understanding how proteins fold and the computational difficulty of playing games. He received the MacArthur Fellowship for his work in computational geometry. He and his father Martin Demaine have produced numerous curved-crease sculptures that explore the intersection of science and art — and that are currently in the Museum of Modern Art in New York.
A Department of Electrical Engineering and Computer Science (EECS) professor whose research spans video graphics and photo-generation, Durand was selected for contributions to computational photography and computer graphics rendering. He also works to develop new algorithms to enable image enhancements and improved scene understanding. He received the ACM SIGGRAPH Computer Graphics Achievement Award in 2016.
Freeman is the Thomas and Gerd Perkins Professor of EECS at MIT. He was selected as a fellow for his contributions to computer vision, machine learning, and computer graphics. His research interests also include Bayesian models of visual perception and computational photography. He received “Outstanding Paper” awards at computer vision and machine learning conferences in 1997, 2006, 2009 and 2012, as well as ACM’s “Test of Time” awards for papers from 1990 and 1995.
Jackson is an EECS professor and associate director of CSAIL whose work has focused on improving the functionality and dependability of software through lightweight formal methods. He was selected by ACM for contributions to software modeling and the creation of Alloy, a modeling language that has been used to find flaws in many designs and protocols. He is a MacVicar Fellow and also received this year's ACM SIGSOFT Impact Paper Award.
ACM will formally recognize the fellows at its annual awards banquet in San Francisco in late June 2017.
Read this article on MIT News.
News Image:

Labs:
Palacios elected IEEE fellow
Department of Electrical Engineering and Computer Science
Tomás Palacios, professor of electrical engineering, recognized for work with semiconductors.
Tomás Palacios, professor of electrical engineering, has been elected as a Fellow of the Institute of Electrical and Electronic Engineers for his “contributions to gallium nitride electron devices and two-dimensional materials.”
IEEE fellows are voted into the fellowship for achieving “an extraordinary record of accomplishments in any of the IEEE fields of interest.” The fellowship is reserved for only 0.1% of the total voting IEEE membership.
At MIT, Palacios leads a research group within the Microsystems Technology Laboratories focused on advanced electronic devices based on new semiconductors, particularly graphene and Gallium Nitride (GaN). Electronic circuits using these “extreme materials” could reduce energy consumption as well as help computers run faster. The Palacios group was the first one in the world to incorporate graphene in a communication system, and it has pioneered the use of graphene and other one-atom-thick materials in high frequency and large area electronics. The Palacios group is also well known in the field of GaN electronics, where it has pushed the state-of-the-art in power amplifier performance and linearity through new transistor designs and its integration with Silicon electronics. Cambridge Electronics, Inc., was founded in 2012 by his former students and postdocs to exploit the commercial applications of some of these technologies.
In recognition of his work, he has also received the Presidential Early Career Award for Scientists and Engineers (PECASE), the Young Investigator Award of the International Symposium on Compound Semiconductors (ISCS), the NSF CAREER Award, the ONR Young Investigator Award, the DARPA Young Faculty Award, and more than ten best paper awards including the Best Paper Award at the International Electron Device Meeting (IEDM), the George Smith Best Paper Award of IEEE Electron Device Letters, and the Device Research Conference. Palacios is also the director of the VI-A Master of Engineering Thesis Program.
Labs:
Bots, Bit Flips, and Catching the Bus
Alison F. Takemura | Department of Electrical Engineering and Computer Science
Engineering undergrads showcase their research after one semester of year-long SuperUROP projects.
151 SuperUROP students presented at the December 8 Research Review. Photo by Gretchen Ertl.
Imagine you’re walking in your dimly lit hallway. You’ve donned a pair of glasses that augment your reality. But the new object in your environment – a sleeping dragon the size of a cat – looks disappointingly flat and cartoonish.
“You can tell it’s really fake, because the lighting [on it] doesn’t match your environment,” explains Elisa Young. A senior in Electrical Engineering and Computer Science (EECS), Young is researching how to make simulated objects reflect the light in a user’s environment. That way, they could look more like objects in the real world.
“I have such love for visual things in combination with computer science,” says Young, enthused to erode the boundary between virtual and concrete realities. She smiles at the thought. “It’s really cool to be like, ‘Oh, I’m living in a movie.’”
Young was among 151 SuperUROP students presenting their work on Thursday, Dec. 8 at the SuperUROP Research Review. The students enrolled in the School of Engineering program, who undertake year-long research projects, shared their first term’s progress with faculty and graduate student mentors.
The relevance of the students’ work — research to enable faster commutes, use robots to help people, optimize buildings, and bolster defenses against massive cyber attacks — made an impression. “It’s amazing to see them tackling these tough problems,” said Anantha Chandrakasan, head of the department of Electrical Engineering and Computer Science. Chandrakasan created the program in 2012 to provide students with an immersive, graduate level research experience.
Cooper Sloan wants to make catching the bus easier using machine learning, a technique that allows computers to pick out subtle patterns. Using the last 5 years of GPS data from Boston buses, Sloan is unraveling the dynamics of the bus system, which has some quirks. “Slow buses tend to get slower and fast buses tend to get faster, which results in clumping,” says the EECS senior. Training a neural net, the architecture by which machines learn, will enable software to better predict bus arrival times—something commuters are waiting for.
Machines could also better assist people right in their homes, including senior citizens who have trouble walking. To that end, Alex LaGrassa is training robots to understand human speech. Right now, robots can easily act on pre-programmed information, she explains: for example, grab the Blue Ribbon muffin mix. Yet in the flurry of a real kitchen, a person might say grab the blue box—using a description rather than a proper name. An EECS junior, LaGrassa is programming a robot to understand natural speech and actively search for objects that meet the speaker’s criteria. “It has that extra step of figuring out ‘What is this person talking about?’,” she says. Having robots bridge that linguistic divide between human and machine would be helpful, says LaGrassa, “Because, you know, requiring people to know how to program is problematic.”
Brenda Stern, a senior in Civil and Environmental Engineering, is looking to design buildings with a smaller carbon footprint. Most people of think of the energy consumed during construction or once a building is up and running. But Stern focuses on the energy that went into creating the building materials—like the concrete or steel columns, slabs, and framework. They all have embodied carbon, or the carbon emissions associated with their production. “I’m finding ways to minimize these materials to create more sustainable structures,” she says.
Valerie Sarge is working on hardware, trying to do more with less data. An EECS junior, Sarge is researching how to transform low-resolution images into high-resolution facsimiles. By using field-programmable gate arrays, or FPGAs, she can program hardware with a neural net to extrapolate information, mimicking the way a human would fill in the dots: “We quickly hallucinate the information that your brain expects to see.” That means you could download a low resolution video onto your phone, and watch it in HD.
The goal is to push current limits of computing power by moving some of the computational demand from software into hardware. If scientists can achieve that, Sarge says, “every type of research, every type of analysis, every type of study that requires computing power — will become easier.”
Another SuperUROP is preparing hardware for space. Madeleine Waller, an EECS senior, is running tests to make the Transiting Exoplanet Survey Satellite, scheduled for launch December 2017, more resilient.
The satellite will contend with meddlesome cosmic rays, which can cause computer chip bits to flip, creating errors in the data. Waller is testing the satellite’s FPGA by intentionally injecting errors into a model data stream. “We’re trying to trigger all the watchdog protocols in the system,” she says. Ensuring they work would give researchers a better chance of confidently detecting exoplanets.
But while some threats to systems are random, others are maliciously calculated. In October, Hyunjoon Song, a senior in EECS, saw news that millions of infected bots had attacked Akamai, a network server company. The strike had wielded an army of “Internet of Things” devices, such as digital cameras and video recorders. Collectively dubbed the Mirai botnet, the bots battered Akamai with 620 Gb per second to attempt to overload the company's servers in what's known as a distributed denial of service (DDoS) attack. “This was one of the most powerful DDoS attacks ever,” he says. “And the problem is that there are billions more of these devices that are just as vulnerable. The Mirai botnet is just the beginning.”
Song is looking at the source code and interacting with the Mirai botnet to understand its architecture and sift for vulnerabilities, in the hope of preventing more serious attacks.
“The students show incredible enthusiasm for their work,” Chandrakasan says. “I’m eager to see how their projects develop over the spring semester.”
News Image:
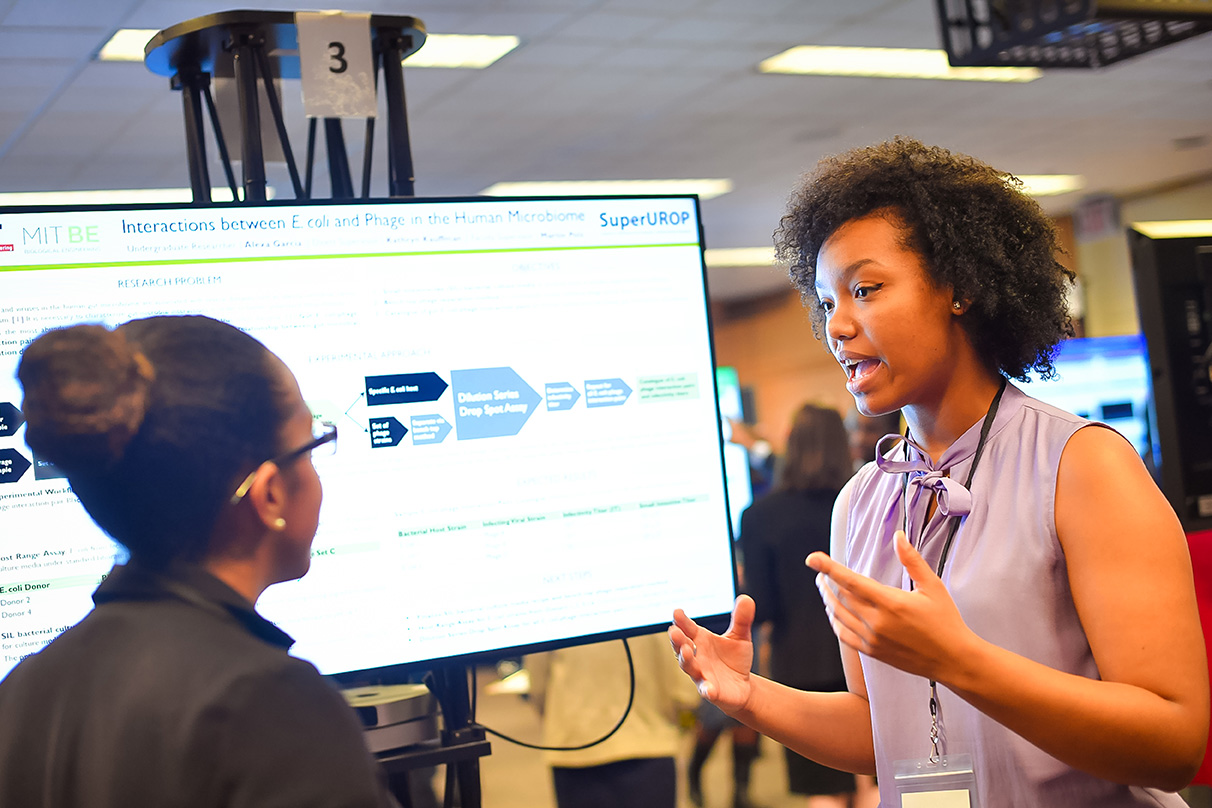
Stefanie Mueller and Justin Solomon included in the "Forbes 30 Under 30" 2017 list.
Two EECS faculty members were honored in the Forbes 30 Under 30 in Science 2017 list that showcases America’s most important young scientists, entrepreneurs, thinkers, and leaders.
Stefanie Mueller, Assistant Professor in EECS as of January 2017, focuses on the computer science of “physical Data,” such as that involved in 3D printing. She is the conference General Co-Chair at the ACM SIGGRAPH Symposium on Computational Fabrication 2017. Mueller received her PhD in human-computer interaction (HCI) from the Hasso Plattner Institute in 2016, where she also received an MS in IT-systems engineering. In her research, Mueller develops novel interactive hardware and software systems that advance personal fabrication technologies. Her work has been published at the most selective HCI venues — Association for Computing Machinery (ACM), the Conference for Human Factors in Computing Systems (CHI), and User Interface Software and Technology (UIST) — and received a best paper award and two best-paper nominees. Mueller is an associate chair of the program committees at ACM, CHI, and UIST, and is a general co-chair for the ACM SIGGRAPH Symposium on Computational Fabrication that will take place at MIT in June 2017. She has been an invited speaker at MIT, Stanford, the University of California at Berkeley, Harvard, Carnegie Mellon University, Cornell University, Microsoft Research, Disney Research, Adobe Research, and others. In addition, her work has been covered widely in New Scientist, BBC, The Atlantic, and The Guardian. Mueller will head the HCI engineering group at MIT's Computer Science and Artificial Intelligence Laboratory, which works at the intersection of human-computer interaction, computer graphics, computer vision, and robotics.
Justin Solomon, Assistant Professor in EECS, researches geometric problems in computer graphics, computer vision and machine learning. Co-inventor of three patents, he also spent five years doing computer research for Pixar, developing new algorithms for image processing in movies. Solomon earned his MS and PhD in computer science from Stanford University, where he also earned a BS in mathematics and computer science. Solomon is a past recipient of the Hertz Foundation fellowship, a National Science Foundation graduate fellowship, and the National Defense science and engineering graduate fellowship. His research focuses on geometric problems appearing in shape analysis, optimization, and data processing, with application in computer graphics, medical imaging, machine learning, and other areas. He taught classes on numerical analysis, computational differential geometry, and computer science at Stanford. His textbook, Numerical Algorithms, was released in 2015 (CRC Press).
Read the full list of Forbes 30 Under 30 in Science 2017.
Date Posted:
Labs:
Card Title Color:
Card Description:
Photo:
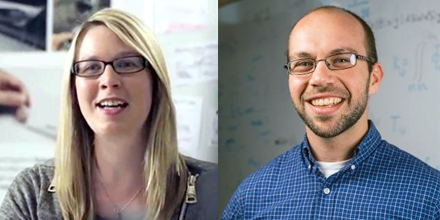
Card Wide Image:

Card Title:
StartMIT: A Workshop for Entrepreneurs and Innovators

Card Wide Image:
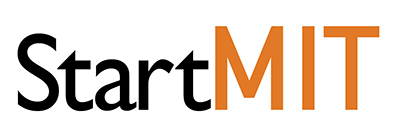
Jobs at EECS
Card Title:
Card URL:
Card Title Color:
StartMIT: A Workshop for Entrepreneurs and Innovators
StartMIT, a class held from January 9 to 23, connects MIT undergrads, grad students, postdocs, and other members of the MIT community with successful entrepreneurs for a crash course on the many paths to entrepreneurship. MIT students will be working closely with entrepreneurs and innovators to develop their ideas; participate in activities to refine their projects, and attend events with alumni and other leaders in the Boston entrepreneurial ecosystem.
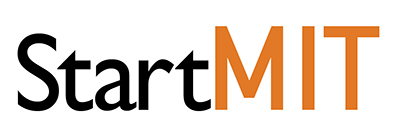
Card Wide Image:
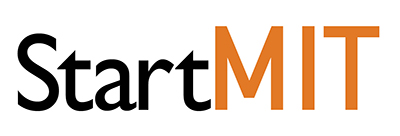
Two from MIT share National Academy of Engineering Fritz J. and Dolores H. Russ Prize
The National Academy of Engineering (NAE) 2017 Fritz J. and Dolores H. Russ Prize was awarded to a team of researchers, including two MIT affiliates, for their work in optical coherence tomography. The award committee noted that the research demonstrated “creative engineering to invent imaging technology essential for preventing blindness and treating vascular and other diseases.”
The Russ Prize, established in October of 1999, recognizes an outstanding bioengineering achievement in widespread use that improves the human condition as well as acknowledges insights that help the public better understand and appreciate the contributions of engineers to health, well-being and quality of life. The Russ Prize, the Gordon Prize, and the Draper Prize, all awarded by the NAE, are considered by many to be, collectively, the "Nobel Prizes of Engineering.”
Sharing the $500,000 Russ Prize were James Fujimoto and Eric Swanson, both of MIT.
James Fujimoto is the Elihu Thomson Professor of Electrical Engineering and Computer Science in the Department of Electrical Engineering and Computer Science (EECS), a visiting professor of ophthalmology at Tufts University School of Medicine, and an adjunct professor at the Medical University of Vienna. His group and collaborators were responsible for the invention and development of optical coherence tomography (OCT). Their paper, “Optical Coherence Tomography,” published in Science in 1991, remains one of the most cited papers in the biophotonics field. Working with Carmen Puliafito and Eric Swanson, he was a cofounder of the startup company Advanced Ophthalmic Devices, which developed OCT for ophthalmic imaging and was acquired by Carl Zeiss. He also cofounded, with Eric Swanson and Mark Brezinski, LightLab Imaging, which developed cardiovascular OCT and was acquired by Goodman, Ltd. and St. Jude Medical.
Eric Swanson SM '84 is an active participant in a variety of entrepreneurial, industrial, academic, and volunteer activities. He chairs the board of directors for Acacia Communications and is a member of the boards of directors for NinePoint Medical and Curata; serves on the governing board of the Danish National Quantum Innovation Center; and is an affiliate of the MIT Deshpande Center for Entrepreneurship and the MIT Translational Fellows Program. He is a cofounder or founding board member of five startup companies: Advanced Ophthalmic Devices, LightLab Imaging, Sycamore Networks, Acacia Communication, and Curata. Swanson worked at MIT Lincoln Laboratory for 16 years, where he collaborated in the discovery and advancement of OCT and worked on optical networks, including one of the first wavelength division multiplexed all-optical networks, and space communication, including one of the first intersatellite laser communication systems.
Complete information on this year’s and past years' Russ Prizes can be found at the National Academy of Engineering website.
See also the MIT News Office article.
Date Posted:
Card Title Color:
Card Description:
Photo:
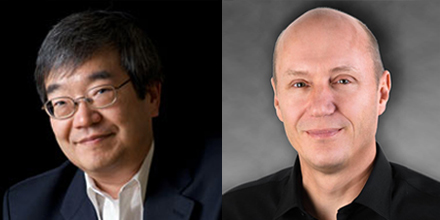
Card Wide Image:
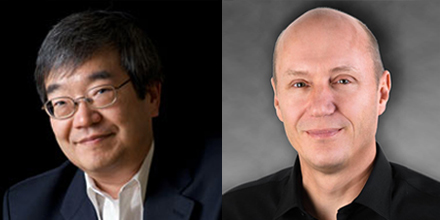
Card Title:
StartMIT’s Innovation Night: Innovators from a range of fields discuss their pioneering work and their founders’ journey.
Innovators in the fields of materials chemistry, online care, natural language processing, social robotics, and venture capital shared their founders’ journey and how they made impact along the way during a panel discussion at StartMIT’s Innovation Night on Jan. 11 at the MIT Media Lab.
Led by moderator Kym McNicholas, an Emmy Award-winning anchor/reporter/producer and entrepreneur, the cast included MIT faculty members Regina Barzilay, the Delta Electronics Professor of Electrical Engineering and Computer Science (EECS); Angela Belcher, the James Mason Crafts Professor in the Department of Materials Science and Engineering and the Department of Biological Engineering; and Cynthia Breazeal, associate professor of media arts and sciences; as well as Donna Levin, co-founder of Care.com, entrepreneur-in-residence at the Martin Trust Center for Entrepreneurship, and lecturer at MIT Sloan School of Management; and Katie Rae, founder and general partner at Project 11 Ventures.
Common themes of passion and timing emerged throughout the conversation as McNicholas asked the panel about topics related to risk, research, inspiration, and failure.

Card Wide Image:

StartMIT’s Innovation Night: Innovators from a range of fields discuss their pioneering work and their founders’ journey.
Innovators in the fields of materials chemistry, online care, natural language processing, social robotics, and venture capital shared their founders’ journey and how they made impact along the way during a panel discussion at StartMIT’s Innovation Night on Jan. 11 at the MIT Media Lab. Led by moderator Kym McNicholas, the cast included MIT faculty members Regina Barzilay, Angela Belcher, and Cynthia Breazeal; as well as Donna Levin, and Katie Rae. Common themes of passion and timing emerged throughout the conversation as McNicholas asked the panel about topics related to risk, research, inspiration, and failure.
Card Wide Image:

EECS Professors Heldt, Madry, Sanchez, and Sze Promoted
MIT Electrical Engineering and Computer Science Department Head Anantha Chandrakasan and Associate Department Heads Nancy Lynch, Asu Ozdaglar, and David Perreault announced in February 2017, the promotions of four faculty members in the department. Professors Thomas Heldt, Aleksander Madry, Daniel Sanchez, and Vivienne Sze are promoted to the rank of Associate Professor without Tenure, effective July 1, 2017.
Prof. Heldt explores the collection and use of high-resolution clinical data and mechanistic modeling of human physiology to improve the diagnosis and treatment of critically ill patients. His research at MIT is forging new and promising directions that will enable personalized health care. An example of Thomas’ ingenuity can be found in his recent work on the non-invasive measurement of intracranial pressure (ICP). Thomas developed a surprisingly robust physiological model and measurement approach that enables ICP to be very accurately estimated using only measurements that can be made non-invasively in the ICU. This new approach, which is already under clinical testing in three Boston hospitals, promises to have worldwide impact in reducing the substantial risk and trauma associated with existing ICP measurement methods. In addition to being an innovative scholar, Thomas is an exceptional educator, and has contributed to courses including Quantitative Systems Physiology (6.022J/HST 542), Cellular Biophysics (6.021J/HST 541) and Introduction to Communication, Control, and Signal Processing (6.011). In recognition of his tremendous strength as an educator, Thomas was awarded the Louis Smullin Prize for Teaching Excellence in 2015.
Prof. Madry works on designing efficient algorithms for fundamental optimization problems. His particular focus is on core graph problems, such as finding maximum flows and minimum cuts, computing matchings, and finding optimal routes. In many cases, he has improved upon decades-old bounds. A common theme behind much of his work is combining continuous optimization techniques, such as gradient descent, interior point methods, and linear system solving, with purely combinatorial techniques. Aleksander's work was recognized with four best paper awards at top conferences in theoretical computer science, honorable mention in the ACM Doctoral Dissertation competition, an NSF CAREER award, and a Sloan fellowship. He has developed a new graduate course on Graph Algorithms and Optimization, and has co-taught the Advanced Algorithms (6.854) and the Introduction to Algorithms (6.006) courses. Aleksander takes active role in organizing activities in the Theory of Computation group, and has served on several important departmental committees. He has also performed extensive service for the broader research community, including co-chairing a semestral program on continuous and discrete optimization at the Simons Institute for Theory of Computing, and co-founding the Interest Group on Algorithmic Foundations of Information Technology (IGAFIT) and Highlights of Algorithms (HALG) conference.
Prof. Sanchez designs efficient, sophisticated architectures for multicore computers, using analytical approaches. His work has addressed many issues involved in designing efficient parallel architectures, most importantly, design of highly efficient caching systems for multiprocessors. He has supervised many other projects containing innovative ideas, such as his COUP project, which exploits commutativity properties of operations to speed up processing in cache-coherent systems. His recent Swarm project develops a new model of multiprocessor computation, based on identifying dependencies between operations and exploiting this information to parallelize program execution. Daniel has won an NSF CAREER award, a Best Paper award for his commutativity work, and two “Micro Top Picks” awards for his work on caching and on Swarm. In addition, one of his PhD students, Nathan Beckmann, finished in 2015 and won the Sprowls award for outstanding PhD thesis in Computer Science. Daniel has taught our major courses in Computation Structures and Computer System Architecture, and earned high teaching ratings in both. He has also developed an Advanced Topics course for computer architecture. He has performed extensive service for the research community and for EECS.
Prof. Sze’s efforts focus on algorithms, circuits and systems for video coding, computer vision and machine learning – applications which are becoming extremely important to our information infrastructure. Vivienne develops new techniques that bridge algorithms and hardware design for these applications, enabling designs optimized across multiple layers of abstraction for greatly improved performance. Examples of her groundbreaking work include customized accelerators that provide substantial power reduction in computer vision and deep learning applications and new video coding algorithms that provide order-of-magnitude throughput improvements. She is widely recognized for her leading work in these areas, and has received many awards, including the DARPA Young Faculty Award, the 3M Corporation Non-Tenured Faculty Award and the AFOSR Young Investigator Award. Vivienne co-edited the book High Efficiency Video Coding (HEVC): Algorithms and Architectures. Her curricular contributions include the introduction of valuable new material into classes including 6.344, Digital Image Processing, and 6.374, Analysis and Design of Digital Integrated Circuits.
Date Posted:
Announcement Sources:
Research Theme:
Labs:
Card Title Color:
Card Description:
Card Wide Image:

Card Title:
Secure wireless chargers
Larry Hardesty | MIT News
New chip would thwart the counterfeiting that plagues the market for wired device chargers.
Counterfeit chargers for portable electronics are a major problem. At the end of 2016, Apple claimed that of 100 Apple-branded charging accessories it bought on Amazon, 90 were counterfeits. Around the same time, Britain’s Chartered Trading Standards Institute reported that of 400 counterfeit chargers it bought from a range of online retailers, 397 failed a basic safety test.
In the last few years, portable electronics that can be recharged wirelessly have started coming to market. In an effort to get ahead of the problem of counterfeit wireless chargers — which could cause power surges that fry a device’s circuitry — researchers from MIT’s Microsystems Technology Laboratories have built a chip that blocks attempts to wirelessly charge a device’s battery unless the charger first provides cryptographic authentication.
The same technology also solves another problem with wireless chargers. When two devices share a single charger, if they are different distances from the charger’s electrical coil, their charging rates can vary enormously, to the extent that one device might charge fully while the other remains virtually uncharged. In the same way that the researchers’ chip can block power transfer from an unauthorized charger, it can slow the power transfer to a device nearer the charging coil, ensuring more equitable charge rates.
“Security is one of the most critical issues in the ‘internet of things [IoT],’” says Anantha Chandrakasan, the Vannevar Bush Professor of Electrical Engineering and Computer Science, referring to the popular idea that vehicles, appliances, civil-engineering structures, manufacturing equipment, and even livestock will soon have sensors that report information directly to networked servers. “We will see security functionality embedded into virtually every function and component of an IoT node.”
The researchers presented the new chip this week at the International Solid-State Circuits Conference. Chandrakasan is the senior author on the conference paper, and the first author is Nachiket Desai, who was an MIT graduate student in electrical engineering and computer science (EECS) when the work was done. They’re joined by Chiraag Juvekar, also an EECS graduate student at MIT, and Shubham Chandak, a graduate student in electrical engineering at Stanford University.
Switched out
In a wireless charging system, both the charger and the target device contain metal coils. An alternating current — an electrical current that changes direction at a regular rate — passing through the charger’s coil produces a magnetic field, which induces a current in the device’s coil. The rate at which the current in the charger alternates defines a frequency, much like the frequency of a radio transmission. The device’s coil must be “tuned” to the transmission frequency in order to receive power.
The MIT researchers’ chief innovation is a more compact and efficient circuit for tuning the frequency of the receiving coil. A standard tuning circuit connects the coil to a series of capacitors, electronic components that can store charge. Between each pair of capacitors is a switch, and switching capacitors on and off changes the receiver’s frequency.
“Those switches have very severe requirements,” Juvekar says. “They either have to block a very large voltage when they’re off, or they have to carry a very large current when they’re on, or in some cases both. If a switch needs to block a very big voltage, then it’s very hard to put that on the chip. So it has to be a discrete component on the [circuit] board, outside the chip. Or if it’s on the chip, it requires a specialized [manufacturing] process that might be very expensive.”
Instead of a single coil attached to a bank of capacitors, the MIT researchers’ design uses a pair of coils attached to one capacitor each — no switches required. “The fact that those switches aren’t there anymore is a big advantage,” Juvekar says.
Tuned in
In the researchers’ chip, one of the coils — the main coil — is much larger than the other — the auxiliary coil. The main coil carries the chief responsibility for charging a device’s battery. When a current is flowing through the auxiliary coil, it produces a magnetic field that changes the tuning frequency of the main coil.
In the circuit connected to the auxiliary coil, the resistance — the efficiency with which it conducts electricity — can be continuously varied. When the resistance is low, the auxiliary coil produces a strong magnetic field, which changes the main coil’s tuning frequency so drastically that charging is impossible.
When the resistance in the auxiliary coil’s circuit is higher, the magnetic field is weaker, and the detuning is less drastic. Some power transfer will still occur, but the charge rate is lower. That permits other, more distant devices to harvest more of the power transmitted by the charger coil.
The chip uses an authentication technique called elliptic curve cryptography, which is a “public-key” cryptographic technique. Using publicly available information, the chip can generate — and verify the response to — a question that only a charger with valid private information can answer. The chip doesn’t need to store a secret key of its own.
Elliptic curve cryptography is a well-established technique. But Chandrakasan’s group has developed a battery of methods for reducing chips’ power consumption, and the researchers found a way to simplify the encryption circuit so that it takes up less space on the chip and consumes less power.
“This paper describes an innovative approach to accurately and securely managing more than one wireless charging load,” says Baher Haroun, director of signal-path research at Texas Instruments’ Kilby Labs. “The need for security in wireless energy distribution is critical to ensure authorized and efficient use of the energy delivered. This work could have benefits for safety but also for [determining] ‘Who is a legitimate user for this delivered energy?’”
The work was sponsored by MIT Lincoln Labs, Texas Instruments, and the Taiwan Semiconductor Manufacturing Company, which manufactured the prototype chips through its University Shuttle program.
Read this article on MIT News
News Image:

Labs:
Dina Katabi among eight MIT faculty elected to the National Academy of Engineering
Eight MIT faculty are among the 84 new members and 22 foreign associates elected to the National Academy of Engineering. Newly elected members for this year also include an impressive 18 MIT-affiliated alumni.
Election to the National Academy of Engineering (NAE) is among the highest professional distinctions accorded to an engineer. Academy membership honors those who have made outstanding contributions to "engineering research, practice, or education, including, where appropriate, significant contributions to the engineering literature," and to "the pioneering of new and developing fields of technology, making major advancements in traditional fields of engineering, or developing/implementing innovative approaches to engineering education."
The eight elected this year include:
Paula Hammond, the David H. Koch Professor, head of the Department of Chemical Engineering, and member of the Koch Institute for Cancer Research, for contributions to self-assembly of polyelectrolytes, colloids, and block copolymers at surfaces and interfaces for energy and health care applications.
Daniel Hastings, the Cecil and Ida Green Education Professor in the Department of Aeronautics and Astronautics and chief executive officer and director of the Singapore-MIT Alliance for Research and Technology, for contributions in spacecraft and space system-environment interactions, space system architecture, and leadership in aerospace research and education.
Dara Entekhabi, the Bacardi and Stockholm Water Foundations Professor in the departments of Civil and Environmental Engineering and Earth, Atmospheric and Planetary Sciences, for leadership in the hydrologic sciences including the scientific underpinnings for satellite observation of the Earth's water cycle.
Dina Katabi, the Andrew (1956) and Erna Viterbi Professor in the Department of Electrical Engineering and Computer Science and a member of the Computer Science and Artificial Intelligence Laboratory (CSAIL), for contributions to network congestion control and to wireless communications.
Alexander H. Slocum, the Pappalardo Professor of Mechanical Engineering in the Department of Mechanical Engineering, for contributions to precision machine design and manufacturing across multiple industries and leadership in engineering education.
Michael S. Strano, the Carbon P. Dubbs Professor of Chemical Engineering in the Department of Chemical Engineering, for contributions to nanotechnology, including fluorescent sensors for human health and solar and thermal energy devices.
Mehmet Toner, professor of health sciences at the Harvard-MIT Division of Health Sciences and Technology and the Helen Andrus Benedict Professor of Surgery at Massachusetts General Hospital, for engineering novel microelectromechanical and microfluidic point-of-care devices that improve detection of cancer, prenatal genetic defects, and infectious disease.
Ioannis Yannas, professor of polymer science and engineering in the Department of Mechanical Engineering, for co-developing the first commercially reproducible artificial skin that facilitates new growth, saving the lives of thousands of burn victims.
“This is a great class of new NAE members who are affiliated with MIT,” says Ian A. Waitz, dean of the School of Engineering and the Jerome C. Hunsaker Professor in the Department of Aeronautics and Astronautics. “It is wonderful to see our faculty and alumni being honored by their peers for contributions of the highest level.”
Including this year’s inductees, 142 current MIT faculty and staff are members of the National Academy of Engineering. With this week’s announcement, this brings NAE’s total U.S. membership to 2,281 and the number of foreign members to 249.
Eighteen MIT alumni, including some of the newly elected members listed above, were also named to the NAE this year. They include: Ellen Arruda PhD '92; Aziz Asphahani PhD '75; David Boger ’83; Mark Daskin '74; Bailey Diffie '65; Eric Ducharme SM ’85, ScD ’87; Dara Entekhabi PhD '90; Paula Hammond '84; Daniel Hastings PhD ’80; Dina Katabi SM ’99, PhD ’03; Robert McCabe SM ’81; Alexander Slocum, Jr. ’82; Megan Smith ’86; Darlene Solomon PhD '85; Mehmet Toner SM ’85, PhD '89; George Varghese PhD ’93; Ioannis Yannas SM '59; and Katherine Yelick '82.
Date Posted:
Announcement Sources:
Labs:
Card Title Color:
Card Description:
Photo:

Card Title:
Putting data in the hands of doctors
by Meg Murphy | School of Engineering
Regina Barzilay is working with MIT students and medical doctors in an ambitious bid to revolutionize cancer care. She is relying on a tool largely unrecognized in the oncology world but deeply familiar to hers: machine learning.
Barzilay, the Delta Electronics Professor of Electrical Engineering and Computer Science, was diagnosed with breast cancer in 2014. She soon learned that good data about the disease is hard to find. “You are desperate for information — for data,” she says now. “Should I use this drug or that? Is that treatment best? What are the odds of recurrence? Without reliable empirical evidence, your treatment choices become your own best guesses.”
Across different areas of cancer care — be it diagnosis, treatment, or prevention — the data protocol is similar. Doctors start the process by mapping patient information into structured data by hand, and then run basic statistical analyses to identify correlations. The approach is primitive compared with what is possible in computer science today, Barzilay says.
These kinds of delays and lapses (which are not limited to cancer treatment), can really hamper scientific advances, Barzilay says. For example, 1.7 million people are diagnosed with cancer in the U.S. every year, but only about 3 percent enroll in clinical trials, according to the American Society of Clinical Oncology. Current research practice relies exclusively on data drawn from this tiny fraction of patients. “We need treatment insights from the other 97 percent receiving cancer care,” she says.
To be clear: Barzilay isn’t looking to up-end the way current clinical research is conducted. She just believes that doctors and biologists — and patients — could benefit if she and other data scientists lent them a helping hand. Innovation is needed and the tools are there to be used.
Barzilay has struck up new research collaborations, drawn in MIT students, launched projects with doctors at Massachusetts General Hospital, and begun empowering cancer treatment with the machine learning insight that has already transformed so many areas of modern life.
Machine learning, real people
At the MIT Stata Center, Barzilay, a lively presence, interrupts herself mid-sentence, leaps up from her office couch, and runs off to check on her students.
She returns with a laugh. An undergraduate group is assisting Barzilay with a federal grant application, and they’re down to the wire on the submission deadline. The funds, she says, would enable her to pay the students for their time. Like Barzilay, they are doing much of this research for free, because they believe in its power to do good. “In all my years at MIT I have never seen students get so excited about the research and volunteer so much of their time,” Barzilay says.
At the center of Barzilay’s project is machine learning, or algorithms that learn from data and find insights without being explicitly programmed where to look for them. This tool, just like the ones Amazon, Netflix, and other sites use to track and predict your preferences as a consumer, can make short work of gaining insight into massive quantities of data.
Applying it to patient data can offer tremendous assistance to people who, as Barzilay knows well, really need the help. Today, she says, a woman cannot retrieve answers to simple questions such as: What was the disease progression for women in my age range with the same tumor characteristics?
What a machine can see
Working closely with collaborators Taghian Alphonse, chief of breast radiation oncology at Massachusetts General Hospital (MGH); Kevin Hughes, co-director of the Avon Comprehensive Breast Evaluation Center at MGH; and Constance Lehman, the chief of the breast imaging division at MGH, Barzilay intends to bring data science into clinical research nationwide. But first, she’s content with connecting her world with theirs.
Barzilay’s work in natural language processing (NLP) enables machines to search, summarize, and interpret textual documents, such as those about cancer patients in pathology reports. Using NLP tools, she and her students extracted clinical information from 108,000 reports provided by area hospitals. The database they've created has an accuracy rate of 98 percent. Next she wants to incorporate treatment outcomes into it.
For another study, Barzilay has developed a database that Hughes and his team can use to monitor the development of atypias, which help identify which patients are at risk of developing cancer later in life.
Machines are good at making predictions — “Why not throw all the information you have about a breast cancer patient into a model?” she says — but Barzilay is wary of having the recommendations arrive as highly complex, computational recommendations without explanation. Jointly with Tommi Jaakkola, a professor of electrical engineering and computer science at MIT, and graduate student Tao Lei, she is also developing interpretable neural models that can justify and explain the machine-based predictive reasoning.
Barzilay is also looking at how new tools can help do preventive work. Mammograms contain lots of information that may be hard for a human eye to decipher. Machines can detect subtle changes and are more capable of detecting low-level patterns. Jointly with Lehman and graduate student Nicolas Locascio, Barzilay is applying deep learning for automating analysis of mammogram data. As the first step, they are aiming to compute density and other scores currently derived by radiologists who manually analyze these images. Their ultimate goal is to identify patients who are likely to develop a tumor before it’s even visible on a mammogram, and also to predict which patients are heading toward recurrence after their initial treatment.
Ultimate success, Barzilay says, will involve drawing on computer science in unexpected ways, and pushing it in a variety of new health-related directions.
Outside her door, several of Barzilay’s students are talking ideas, hunching over laptops, and drinking coffee. An object set against the back wall resembles an odd coatrack. Guided by an idea from Taghian, six undergraduate students, led by graduate student Julian Straub, built a device that uses machine-learning to detect lymphedema, a swelling of the extremities that can be caused by the removal of or damage to lymph nodes as part of cancer treatment. It can be disabling and incurable unless detected early. Because of their high cost, these machines — lymphometers — are rare in the U.S.; very few hospitals have them.
Students have created an affordable version. And they hope to start testing this device at MGH in a couple of months. “These students are doing amazing work,” says Barzilay. “These innovations will make a really big difference. It is an entry point. There is so much to do. We are just getting started.”
Date Posted:
Announcement Sources:
Labs:
Card Title Color:
Card Description:
Card Wide Image:
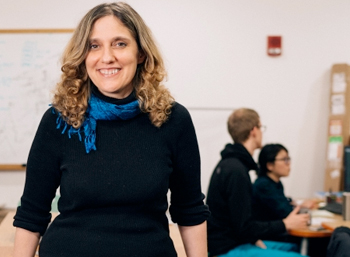
Card Title:
Institute Professor Emerita Mildred Dresselhaus, a pioneer in the electronic properties of materials, dies at 86

Card Wide Image:
