Rachel Gordon | MIT News | CSAIL
“Foundry” tool from the Computer Science and Artificial Intelligence Lab lets you design a wide range of multi-material 3-D-printed objects.
To demonstrate Foundry, MIT researchers designed and fabricated skis with retro-reflective surfaces, a ping-pong paddle, a helmet, and even a bone that may someday be used for surgical planning. Image: Kiril Vidimče/MIT CSAIL
3-D printing has progressed over the last decade to include multi-material fabrication, enabling production of powerful, functional objects. While many advances have been made, it still has been difficult for non-programmers to create objects made of many materials (or mixtures of materials) without a more user-friendly interface.
But this week, a team from MIT's Computer Science and Artificial Intelligence Laboratory (CSAIL) will present “Foundry,” a system for custom-designing a variety of 3-D printed objects with multiple materials.
“In traditional manufacturing, objects made of different materials are manufactured via separate processes and then assembled with an adhesive or another binding process,” says PhD student Kiril Vidimče, who is first author on the paper. “Even existing multi-material 3-D printers have a similar workflow: parts are designed in traditional CAD [computer-aided-design] systems one at a time and then the print software allows the user to assign a single material to each part.”
In contrast, Foundry allows users to vary the material properties at a very fine resolution that hasn’t been possible before.
“It’s like Photoshop for 3-D materials, allowing you to design objects made of new composite materials that have the optimal mechanical, thermal, and conductive properties that you need for a given task,” says Vidimče. “You are only constrained by your creativity and your ideas on how to combine materials in novel ways.”
To demonstrate, the team designed and fabricated a ping-pong paddle, skis with retro-reflective surfaces, a tricycle wheel, a helmet, and even a bone that could someday be used for surgical planning.
Read this article on MIT News or the CSAIL website .
Redesigning multi-material objects in existing design tools would take experienced engineers many days — and some designs would actually be completely infeasible. With Foundry, you can create these designs in minutes. “
3-D printing is about more than just clicking a button and seeing the product,” Vidimče says. “It’s about printing things that can’t currently be made with traditional manufacturing.”
The paper’s co-authors include MIT Professor Wojciech Matusik and students from his Computational Fabrication Group: PhD student Alexandre Kaspar and former graduate student Ye Wang. The paper will be presented later this week at the Association for Computing Machinery’s User Interface Software and Technology Symposium (UIST) in Tokyo.
How it works
Today’s multi-material 3-D printers are mostly used for prototyping, because the materials currently used are not very functional. Users typically create preliminary models, make rapid adjustments, and then print them again. New platforms such as MIT’s MultiFab are developing highly functional materials appropriate for volume manufacturing.
Foundry, meanwhile, serves as the interface to help create such objects. To use it, you first design your object in a traditional CAD package like SolidWorks. Once the file is exported, you can determine the object’s composition by creating an “operator graph” that can include any of approximately 100 fine-tuned actions called “operators.”
Operators can “subdivide,” “remap,” or “assign” materials. Some operators cleanly divide an object into two or more different materials, while others provide more of a gradual shift from one material to another.
Foundry lets you mix and match any combination of materials and also assign specific properties to different parts of the object, combining operators together to make new ones.
For example, if you want to make a cube that is both rigid and elastic, you would assign a “rigid operator” to make one part rigid and an “elastomer operator” to the other part elastic; a third “gradient operator” connects the two and introduces a gradual transition between materials.
Users can preview their design in real-time, rather than having to wait until the final steps in the printing process to see what it will look like.
Testing the system
To test Foundry, the team tried the system on non-designers. They were given three different objects to reproduce: a teddy bear, a bone structure, and an integrated “tweel” (tire and wheel). With just an hour's explanation, users could design the bone, tire wheel, and teddy bear in an average of 56, 48, and 26 minutes, respectively.
In addition to the user study, the team also fabricated a custom wheel for a toddler tricycle. The wheel had an improved structure to maximize lateral strength, and a foam outer wheel for improved suspension.
Using Foundry to exploit the full capabilities of the 3-D printing platform enables many practical applications in medicine and more. Surgeons could create high-quality replicas of objects like bones to practice on, while doctors could also develop more comfortable dentures and other products that would benefit from having both soft and rigid components.
Vidimče’s ultimate dream is for Foundry to create a community of designers who can share new operators with each other to expand the possibilities of what can be produced. He also hopes to integrate Foundry into the workflow of existing CAD systems.
“The user should be able to iterate on the material composition in a similar manner to how they iterate on the geometry of the part being designed,” Vidimče says. “Integrating physics simulations to predict the behavior of the part will allow rapid iteration on the final design.”
The research was supported by the National Science Foundation.
Read this article on MIT News or the CSAIL website.
News Image:
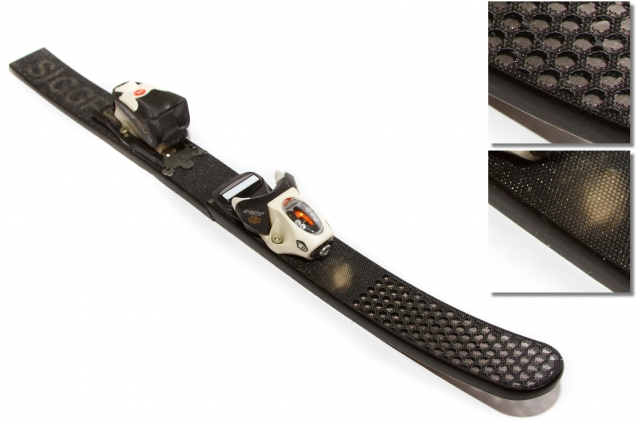